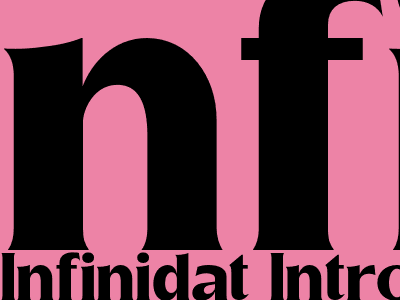
Infinidat Introduces Retrieval-Augmented Generation (RAG) Workflow Deployment Architecture to Make AI More Accurate for Enterprises
Infinidat's Retrieval-Augmented Generation (RAG) Workflow Deployment Architecture
Infinidat, a leading provider of enterprise storage solutions, has introduced Retrieval-Augmented Generation (RAG) Workflow Deployment Architecture. This new architecture is designed to make AI more accurate for enterprises by providing a more efficient and effective way to train AI models.
Traditional AI training methods rely on large amounts of labeled data. This data is used to train the AI model to recognize patterns and make predictions. However, labeling data can be a time-consuming and expensive process. RAG Workflow Deployment Architecture addresses this challenge by using a novel approach that combines retrieval and generation techniques.
How RAG Workflow Deployment Architecture Works
RAG Workflow Deployment Architecture uses two main components: a retrieval module and a generation module.
- The retrieval module searches for relevant data from a large corpus of unlabeled data.
- The generation module then uses the retrieved data to train the AI model.
By combining retrieval and generation, RAG Workflow Deployment Architecture can significantly reduce the amount of labeled data required to train AI models. This makes AI training faster, more cost-effective, and more accurate.
Benefits of RAG Workflow Deployment Architecture
RAG Workflow Deployment Architecture offers a number of benefits for enterprises, including:
- Faster AI training: RAG Workflow Deployment Architecture can significantly reduce the amount of time required to train AI models.
- Reduced costs: RAG Workflow Deployment Architecture can reduce the cost of AI training by reducing the amount of labeled data required.
- Improved accuracy: RAG Workflow Deployment Architecture can improve the accuracy of AI models by providing a more efficient and effective way to train them.
Use Cases for RAG Workflow Deployment Architecture
RAG Workflow Deployment Architecture can be used for a variety of AI applications, including:
- Natural language processing: RAG Workflow Deployment Architecture can be used to train AI models for natural language processing tasks, such as text classification, sentiment analysis, and machine translation.
- Computer vision: RAG Workflow Deployment Architecture can be used to train AI models for computer vision tasks, such as object detection, image classification, and facial recognition.
- Speech recognition: RAG Workflow Deployment Architecture can be used to train AI models for speech recognition tasks, such as voice commands, dictation, and transcription.
Conclusion
Infinidat's Retrieval-Augmented Generation (RAG) Workflow Deployment Architecture is a revolutionary new approach to AI training. This architecture can significantly reduce the amount of labeled data required to train AI models, making AI training faster, more cost-effective, and more accurate. RAG Workflow Deployment Architecture has the potential to transform a wide range of AI applications, from natural language processing to computer vision to speech recognition.